讲座名称:Data Modelling and Analysis using the New Discriminative Broad LearningSystem
讲座时间: 2019-04-26 16:00:00
讲座地点:西电南校区G-420
讲座人:陈俊龙 (C. L. Philip Chen)
讲座人介绍:
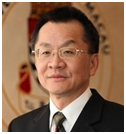
陈俊龙(C. L. Philip Chen),教授,自动化学会副理事长,国家千人学者、国家特聘专家,澳门科协副会长,澳门大学讲座教授,科技学院前院长。陈教授是IEEE Fellow(院/会士)、美国科学促进会AAAS Fellow(院/会士)、国际模式识别IAPRFellow(院/会士)、欧洲科学院院士、欧洲科学与艺术院院士、国际系统及控制论科学院IASCYS院士、自动化学会(CAA)、及香港工程师学会(HKIE) Fellow,现任IEEE系统人机及智能学会的SCI期刊主编(IEEE Trans. on Systems, Man, and Cybernetics: Systems),曾任该学会国际总主席 (2012-2013)。陈教授主要的科研方向包括智能系统与控制,计算智能,混合智能,数据科学,是2018 Clarivate Analytics2018年“计算机科学学科的全球高被引科学家。详见https://orcid.org/0000-0001-5451-7230。2016年他获得了母校,美国普度大学,的杰出电机及计算机工程奖。2018年他获得了IEEE系统科学控制论的最高学术维纳奖(Norbert Wiener Award)。
讲座内容:
Deep learning has carved out a research wave in machine learning. With outstanding performance, more and more applications of deep learning in pattern recognition, image recognition, speech recognition, and video processing have been developed. The talk is to introduce “Broad Learning” – a complete paradigm shift in discriminative learning and a very fast and accurate learning without deep structure. The broad learning system (BLS) utilizes the power of incremental learning. That is without stacking the layer-structure, the designed neural networks expand the neural nodes broadly and update the weights of the neural networks incrementally when additional nodes are needed and when the input data entering to the neural networks continuously. The designed network structure and incremental learning algorithm are perfectly suitable for modeling and learning big data environment. Several BLS variations that cover existing deep-wide/broad-wide structures and their regression performance over function approximation, time series prediction, face recognition, and data modellingwill be discussed.